Welcome to my test page!
How it works
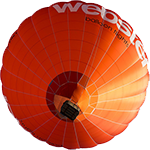
I want to create interactive ipynb visualizations which share and update linked data between cells and persists when converted to html. Jupyter Notebooks being a web app makes this possible as Ipynb's cell outputs are actually just IFRAMES. Jupyter notebooks run a web server responsible for routing communication from your browser to your local machine.
Doing this will store the dataset into the browsers window.
This way, you no longer need to pass the dataset to create Multiple Visualizations.
Similar Tools
Pandoc: A universal document converter. That's it.
quarto: Uses Pandoc to publish webpages with styled flair and web functionality.
nbdev: Focuses as a dev tool but uses quarto for documentation. Previously used a combination of nbconvert and jekyll to get the same effect.
nbconvert: Uses pandoc to publish with styled flair.
Jupyter Book: Creates books from Jupyter Notebooks. Uses nbconvert.
Voilà: Transforms Jupyter notebooks into web applications. Uses nbconvert.
Papermill: Execute and parameterize notebooks. Uses nbconvert.
Jupytext: Pairs notebooks with Markdown or Python scripts. Uses nbconvert.
Sphinx: Generates documentation from source code, not directly focused on Jupyter Notebooks but still relevant for documentation.
Read The Docs: Hosts documentation, integrates with Sphinx and MkDocs.
Other Tools
Docusaurus: Builds optimized websites and supports Markdown, relevant for webpage generation.
MkDocs: Generates documentation from Markdown files, similar in producing web content.
Jekyll: A static site generator, adaptable for documentation, similar in web content generation.
Asciidoctor: Processes AsciiDoc files for documentation, less directly related but still in the realm of web documentation.
How it works
I will first cover basic core functions before showing the convenience function that makes it all easier to use.
Terms:
label - For the dataset
ds - the pands dataframe mapped to the label
vizId - To update the visual
vizType - Specifies the default viz type
rows / cols - To be used on the viztype
To use the tool, first perform typical python data science operations
From there, it is possible to...
Display the data as is. This will visualize the dataframe that is non-updateable
Save the current state of the df to a label and then display it using the label name.
Create a mapping between a label and an elementId so it may be later updated.
Render the mappings
Multiple labels, and mappings to the labels may be made. To update the data and mappings (above), it's just more of the same.
undefined combines the three functions. Toss it w/e - and w/e will update or be made.
Lets create another visual mapping for the existing label
And to create a new saved dataset and visualize in one go we just add the ds
As ininusated earlier, it's possible specify where the visual should be placed by using undefined or magicundefined to render the html div with an id of the vizId specified in your mapping
undefinedAnd now data can be inserted into it. The container can be placed anywhere, but must be executed before the call for visualizing.